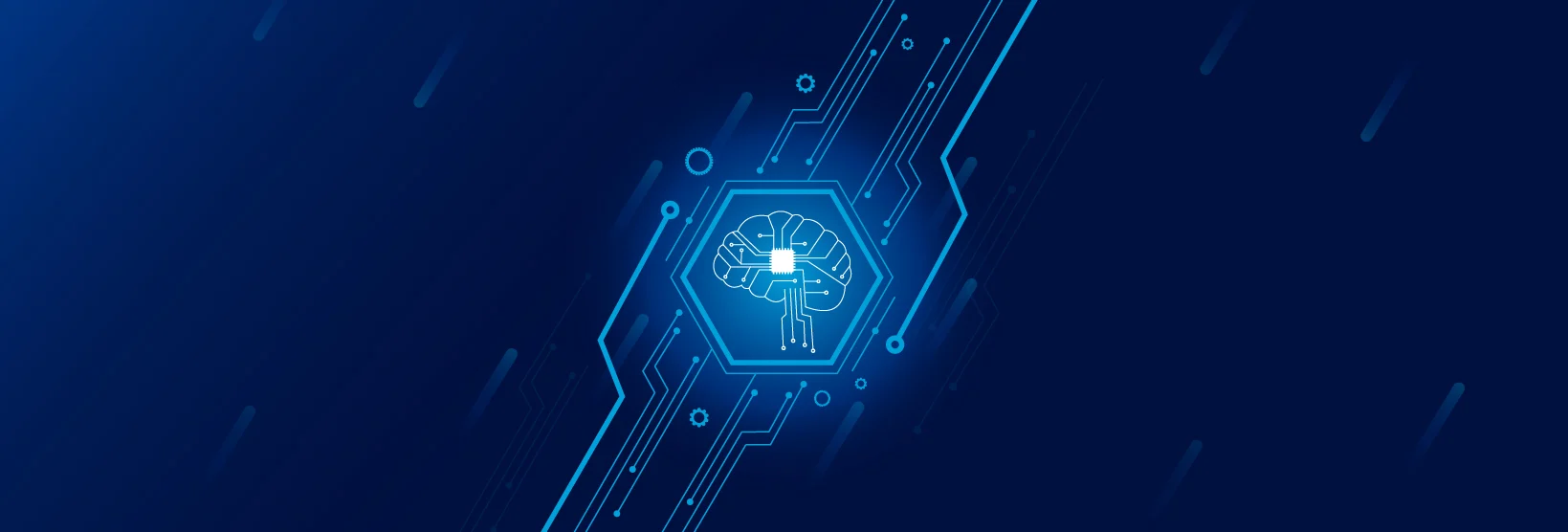
Defining Decision Intelligence
Decision Intelligence (DI) is a discipline that helps answer the question: “What should we do to achieve our desired outcomes?” As DI has evolved multiple definitions of DI have emerged that differ slightly. Originally, the concept emerged as an engineering discipline intended to combine the precision of AI with human intellect. The term “Decision Intelligence” was first coined by Dr. Lorien Pratt, cofounder of Quantellia, popularized in her first book Link and further expanded on in her second book The Decision Intelligence Handbook. DI has now evolved to become a multifaceted concept that functions as a domain (a specific area of application), a discipline (a field of study with its own methodologies and principles), and a framework (a structured approach to enhance decision-making processes). It’s this comprehensive nature that enables Decision Intelligence to effectively contribute to various organizational and business contexts. The DI recipe includes but is not limited to machine learning, big data, intelligence augmentation, predictive analytics, visual analytics, dashboards, complex systems analysis, causal modeling, agency theory, statistical analysis, and more in a way that answers the most practical of all question which is: “If we take these actions today, how will they impact our desired outcomes?”
Decision Intelligence Definitions
The history of Decision Intelligence and it’s 10+ year journey out of the lab can be read on Dr. Pratt’s blog here. Recently it has been defined by various sources, each providing a unique perspective on its nature and applications. Gartner’s definition positions DI as a domain that encompasses decision management, decision support, and various analytics techniques to design, model, align, execute, monitor, and tune decision models and processes. Wikipedia adds depth by considering DI as an engineering discipline that augments data science with theories from social science, decision theory, and managerial science. NTT Data focuses on the human cognitive behavior of decision-making, emphasizing the combination of human intellect and artificial intelligence in achieving specific goals.
Peak AI emphasizes the commercial application of AI to decision-making processes across all business areas. Quantexa, building on Gartner’s definition, highlights DI’s role in understanding and engineering the decision-making process, using artificial intelligence to unlock the power of entity resolution and graph analytics. Tellius describes DI as the application of machine learning and automation to augment human decision-making, facilitating faster and more accurate insights-driven decisions.
Diwo emphasizes DI as a data-driven process enabling faster, more accurate, fact-based decisions. Aera defines DI as the digitization, augmentation, and automation of decision-making across the enterprise, combining AI and machine learning to make faster, more accurate decisions. Infoplus, using a simple yet effective explanation, defines a decision as a choice made after considering various possibilities and highlights AI’s ability to consider all available information objectively.
How Do Decision Models Work?
Decision models form the backbone of Decision Intelligence, utilizing various technologies and algorithms to enhance the decision-making process. Machine learning, as a foundational element, works with structured data to make suggestions or decisions based on specified parameters. Deep learning, a progression from machine learning, considers previous decisions and outcomes when making new suggestions, incorporating a learning element.
Visual decision modeling serves as a bridge between AI decision-making and human decision-makers, providing a visual representation of available options and their potential outcomes. Complex systems modeling enables the rapid construction of intricate business logic based on available data and final goals. Predictive analytics utilizes accurate predictions derived from historical and current data to inform decision-making, as seen in applications like price prediction and automated optimization in retail.
Gartner categorizes Decision Intelligence support into three levels: decision support, decision augmentation, and decision automation. Decision support provides basic tools for human decision-making, while decision augmentation involves proactive machine analysis and recommendations, reviewed and confirmed by humans. Decision automation represents the highest level, where machines autonomously make and execute decisions, with human oversight for risk monitoring and system improvement.
What Decision Intelligence is Not
To fully understand Decision Intelligence, it is essential to differentiate it from other related concepts. Decision Science, as highlighted by Martech.org, is associated with the qualitative side of data, focusing on the operational side, while Decision Intelligence is the operational side itself. It distinguishes Decision Intelligence from strategic intelligence, emphasizing that not every output or recommendation qualifies as a decision.
Aera specifies that Decision Intelligence is not synonymous with process mining, data visualization, or robotic process automation (RPA), as these tools primarily address the speed of legacy processes but do not comprehensively meet the challenges of contemporary business decision-making.
Cognyte provides insights into the differences between Decision Intelligence, Business Intelligence (BI), and Artificial Intelligence (AI). Decision Intelligence merges BI with data science and other disciplines to investigate the “why” behind business performance and facilitate a full-cycle decision process. BI, on the other hand, focuses on visualizing aggregated data, and AI is a broad technology with applications across various fields.
Peak.ai emphasizes that while AI is a theoretical and developmental concept, Decision Intelligence is its practical application in the commercial decision-making process. Tellius differentiates Decision Intelligence from BI, manual analysis (SQL/Python/Excel), and data science tools, highlighting the inclusion of intelligent automation as a key distinguishing factor.
LexisNexis emphasizes the intertwined nature of BI, Decision Intelligence, and AI, clarifying that BI involves data analysis to support informed decisions, AI simulates human intelligence in machines, and Decision Intelligence is specifically molded for decision-making in business contexts.
Xbsoftware.com provides a comprehensive overview of Decision Intelligence in comparison to BI and AI. Business Intelligence involves processes, technologies, and tools for collecting, storing, analyzing, and presenting historical data. Artificial Intelligence aims to create machines capable of tasks requiring human intelligence. Decision Intelligence combines BI and AI, using current or future data to understand actions, outcomes, scenarios, and feedback.