Data Analysis vs. Action: Are You Making the Right Decisions?
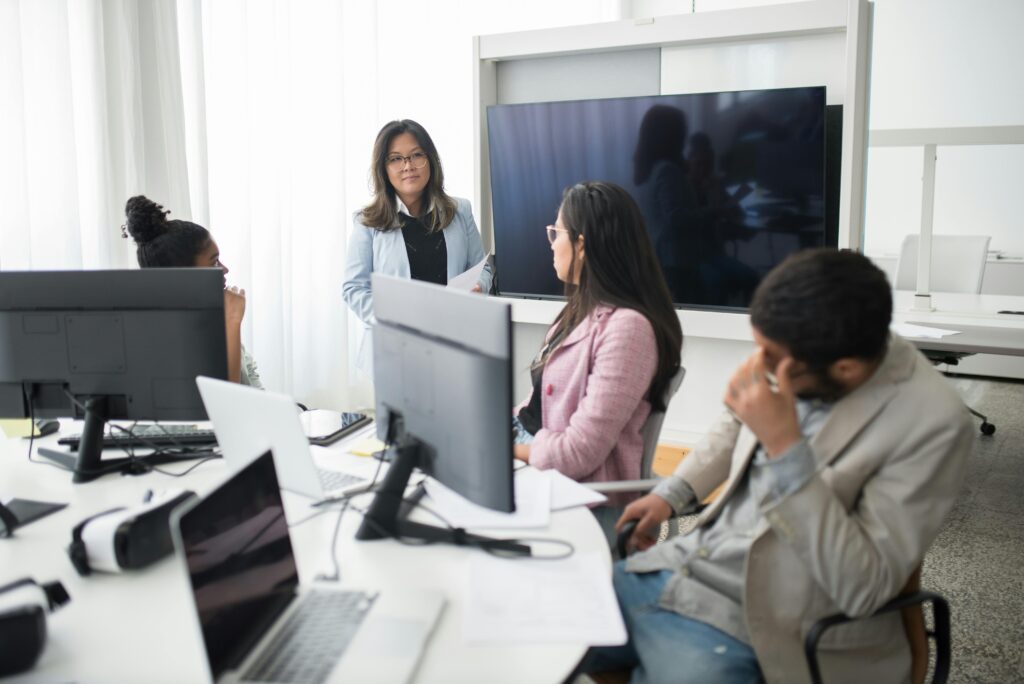
In the evolving landscape of Decision Intelligence (DI), a critical distinction is often overlooked—the difference between making decisions based on data analysis and making decisions to take action in the real world to achieve business outcomes. This nuanced understanding is essential for organizations striving to leverage data effectively to drive strategic success.
Data Analysis and Insight Generation
The initial phase in the DI process involves rigorous data analysis, where organizations use various analytical tools and techniques to extract meaningful insights. This stage usually encompasses:
- Descriptive Analytics: Understanding what has happened in the past.
- Predictive Analytics: Forecasting future trends based on historical data.
- Prescriptive Analytics: Recommending actions based on predictive insights.
These methodologies are invaluable for identifying patterns, anomalies, and trends within vast datasets. However, the true value of these insights is realized only when they inform actionable decisions.
Action-Oriented Decision-Making
The next crucial phase is the application of these insights to real-world scenarios. This involves:
- Strategic Implementation: Developing and executing plans based on data-driven insights.
- Operational Execution: Ensuring that these plans are carried out effectively to achieve desired outcomes.
This transition from analysis to action is where many organizations falter. Insights, no matter how profound, must be operationalized to impact business performance. This involves not only strategic vision but also practical execution.
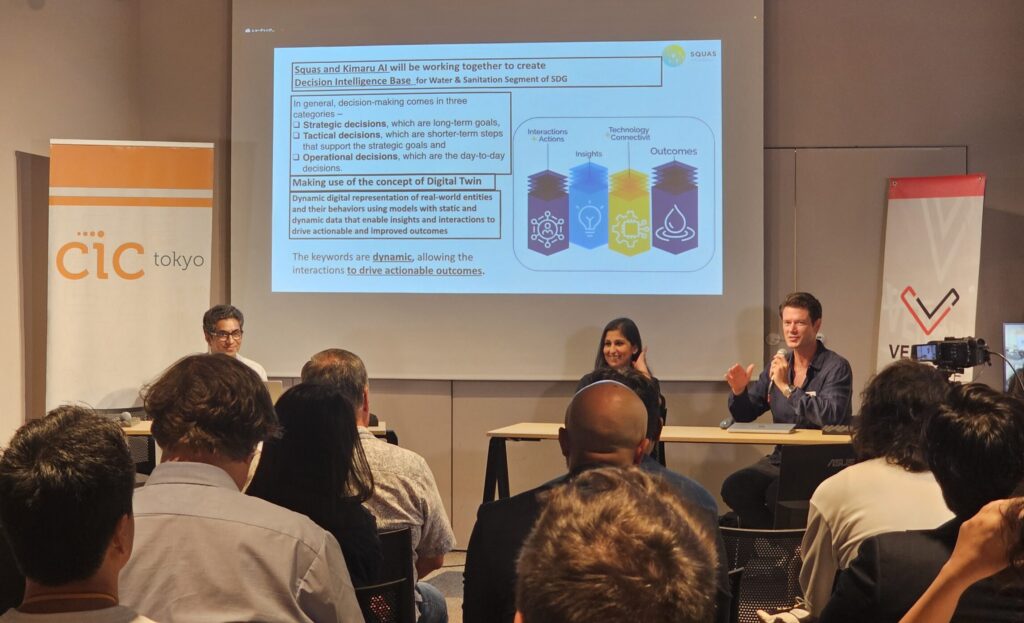
Practical Implications
Consider the following examples to illustrate this distinction, aligned with key Sustainable Development Goals (SDGs):
- Providing Clean Water (SDG 6):
- Insight Generation: Data analysis reveals regions with high contamination levels in water sources.
- Action-Oriented Decision: Implementing water purification projects and infrastructure improvements to ensure access to safe drinking water for affected communities.
- Quality Education (SDG 4):
- Insight Generation: Analysis of educational data highlights areas with low literacy rates and poor school attendance.
- Action-Oriented Decision: Launching targeted educational programs and resource allocation to improve school infrastructure, train teachers, and provide learning materials in underserved areas.
- No Poverty (SDG 1):
- Insight Generation: Socioeconomic data identifies communities with the highest poverty rates and the factors contributing to economic hardship.
- Action-Oriented Decision: Developing and implementing comprehensive poverty alleviation programs, including vocational training, microfinance initiatives, and social safety nets to support economic stability and growth.
These examples underscore the importance of not merely stopping at insight generation but proceeding to the critical phase of implementation and execution.
Also, check out the video of our event where we discussed the uses of Decision Intelligence towards social impact.
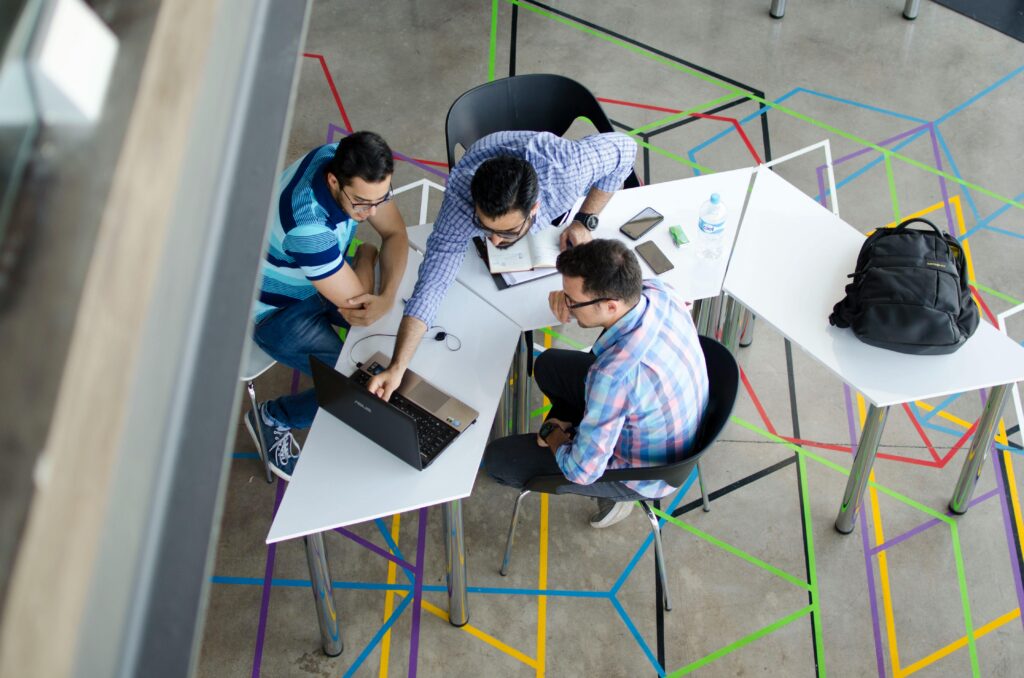
Bridging the Gap
Organizational Context and Execution
Effective implementation of DI requires a nuanced understanding of organizational context. This includes:
- Resource Allocation: Ensuring that the necessary resources—financial, human, and technological—are available to support the execution of data-driven strategies.
- Change Management: Navigating the organizational change that often accompanies new strategic initiatives.
- Performance Measurement: Establishing metrics to assess the impact of implemented strategies and make necessary adjustments.
Educational Imperatives
From an academic perspective, it is imperative that educational programs in Decision Intelligence emphasize this distinction. Students must be equipped not only with the technical skills to analyze data but also with the strategic acumen to translate these insights into actionable decisions. This requires a curriculum that integrates:
- Case Studies: Real-world examples that highlight successful implementations of DI.
- Practical Exercises: Hands-on activities that simulate the transition from data analysis to strategic action.
- Interdisciplinary Approaches: Incorporating insights from business strategy, organizational behavior, and change management.
Check out our list of recommended readings on Decision Intelligence.
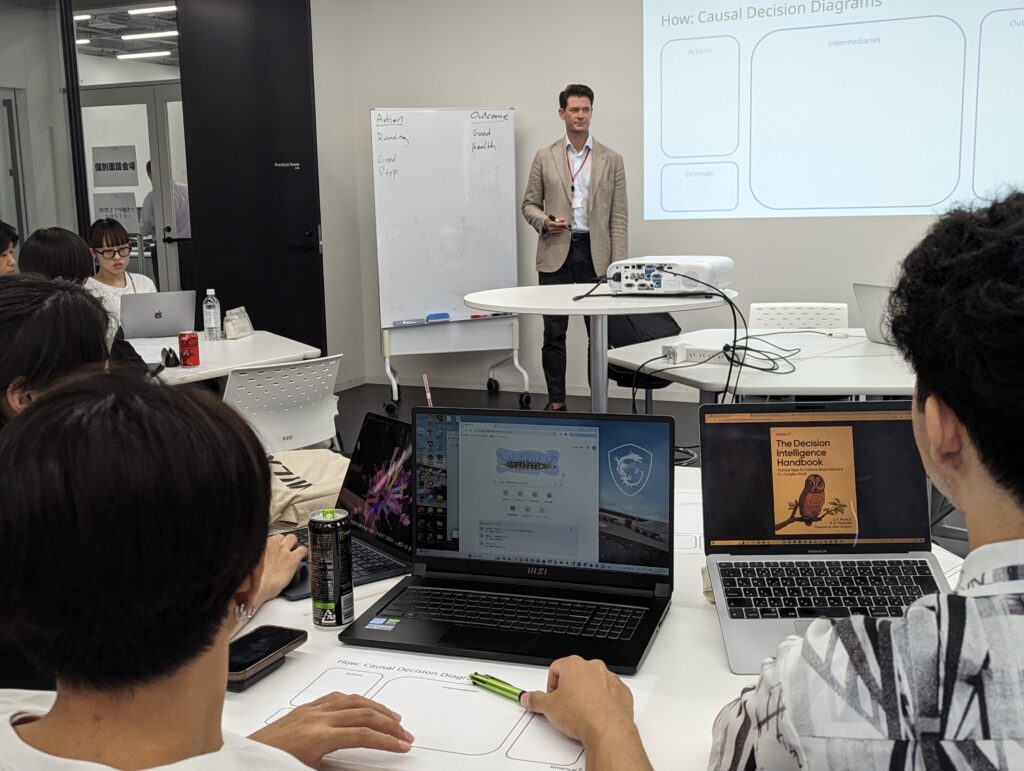
Conclusion
The distinction between data analysis and action-oriented decision-making is fundamental to the effective application of Decision Intelligence. Organizations must strive to bridge this gap to unlock the full potential of their data. By emphasizing both theoretical understanding and practical application, educational institutions can prepare the next generation of professionals to excel in this critical aspect of DI.
As we continue to advance in the field of Decision Intelligence, it is crucial to remember that insights are only as valuable as the actions they inform. Bridging the gap between analysis and action is not just a best practice—it is the cornerstone of strategic success in the data-driven era.
ニュースレターに登録する
kimaru.aiやAI意思決定日本グループの最新情報を受け取るには、ぜひご登録ください。